The Downstream Data Journey
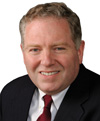
Why does the opportunity to use downstream data significantly outweigh its actual use?
Byrne: Data readiness is the main issue. Adding trade channel data to forecasting involves a magnitude of effort and complexity that is well beyond what most companies can handle to source, cleanse and evaluate the spectrum of data. Even just customer order data is too large for planners to examine all products for all locations daily using manual processes; large consumer products companies have item/location combinations in the tens of thousands. Adding other downstream signals — shipments, retail warehouse withdrawals or point-of-sale (POS) store data — has an exponential impact.
Timeliness is the second issue. The utility of downstream data exists primarily for very near-term planning. Without automated systems having capacity to efficiently process this massive data each day, most of the inherent value is lost.
However, new tools leveraging advanced mathematics and fresh thinking about the forecasting process have enabled some visionary companies to overcome the difficulties. The promise of downstream data can be realized.
What are the different levels of downstream data use?
Byrne: The downstream data maturity model shows how companies improve data leverage by embracing process automation to optimize demand-driven value. The entry point is the demand signal repository (DSR), which enables the manufacturer to observe and report on retailer and consumer demand characteristics based on a variety of syndicated and retailer POS data.
For those venturing beyond observing demand to anticipating supply requirements, the maturity path is more strategic than serial and it is possible to jump directly to the most advanced level.
The developing analytics maturity level is a “point source” approach, employing business intelligence tools to find insights specific to one aspect. It is useful for longer-term strategy development.
The store-level modeling maturity level focuses on store-level analytics. It is helpful to map out-of-stocks but is not scalable.
The enterprise-level supply chain maturity level, tagged “demand sensing”, is a scalable daily forecasting method that relies on mathematical pattern recognition algorithms to parse through all available data to predict orders. It produces visibility that enables the manufacturer to understand and proactively respond to events as they unfold.
How can manufacturers use downstream data to complement lean manufacturing strategies?
Byrne: It’s symbiotic: the better demand signals feed directly into lean manufacturing processes, and lean methodologies are a means to monetize forecast accuracy.
Lean strategy hinges on improving value by eliminating waste. By incorporating downstream data into sales and operations planning, the manufacturer can cut waste by better aligning expected value (forecasted demand) with real value (actual demand).
Demand sensing — tapping customer-facing data from store deliveries to retail promotions to the point-of-sale — leads to a shift to demand-driven operations that extends the manufacturer’s lean process all the way to the retail store level.
Under historical data modeling, forecasts are adjusted as actual orders come in. Adjustments directly reflect how well the future follows the past. With demand sensing, the manufacturer uses an array of daily data to better predict what orders will be for the coming weeks with significantly greater accuracy.
Now the manufacturer can pre-position stock closer to demand points with ample shipping lead times. Expedited rush shipments (which incur a lot of supply chain waste) are avoided. Freight execution can switch modes from more costly trucking to less costly intermodal service. And the waste involved in inventory shrink, obsolescence, repositioning and carrying cost diminishes as the manufacturer optimizes network inventory levels.
And by applying the same discipline to supplier-facing upstream data, the manufacturer can capture more lean value by anticipating inbound materials and services requirements.